#9: Zsolt Olah - Season 1, Episode 10 - Talent is Everywhere!
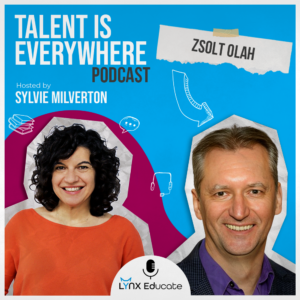
About the Episode:
In this episode of “Talent is Everywhere,” Sylvie Milverton, CEO of Lynx Educate, talks with Zsolt Olah from Intel about the critical role of data in learning and development. Zsolt shares his insights on how learning teams can better interpret and utilize data to enhance outcomes and make strategic decisions.
The discussion highlights the challenges professionals face when working with data, including the importance of questioning assumptions and understanding the story behind the numbers. Zsolt also introduces his interactive workshop at DevLearn, where participants learn to think critically about data and collaborate on making informed decisions.
Transcript
Zsolt:
And that’s that’s exactly the point. So every time you see anything on a chart or you collect data for the first question is why? What are you measuring and why? If you’re not going to make any decisions based on the numbers that whatever the result is, then why you’re measuring the whole thing is basically just waste of time.
Sylvie:
Hi, I’m Sylvie Milverton, CEO of Lynx Educate. This is “Talent is Everywhere”. We’re here to talk about how to keep talent and how to develop talent in order to build a strong business. We’ll interview leaders to hear their best experiences of how they invested in people.
So today we’re going to do a slightly different kind of episode. We’re talking to Zsolt Olah, who works at Intel with a background in data and technology, and he is passionate about working with learning teams to help them understand how to build better learning outcomes, how to create good data and how to make sure learning teams design the right strategy to really interpret, data.
So we’re going to go through a little bit of information, a little exercise around a workshop to see how Zsolt does this. And so thank you for joining me. Why don’t we start with how did you come to this like what do you see as the biggest challenge with data and learning and professionals? And what problem are you trying to solve?
Zsolt:
First of all, thank you for having me. So I’m always happy to talk about data and especially in terms of context of people. and you can see that in my sort of little spiel, I’ve always been the, the bridge between people and, and data. And that’s, that was my call the last four years or so. I spent a couple of years with Amazon and now with Intel.
And what I noticed is that in the HR community, in the learning professionals community, there’s so much enthusiasm, expertise about creativity, helping people grow, gaining skills, that sort of thing. Less enthusiasm about using actual data. And when I talk to people, they all want to to show their values that are business, but they want to jump from knowing nothing about how to speak and use data to ROI calculations.
And when they fail, they jump back to your full back to the original. Or let’s just not do anything. So my goal and my vision is actually starting from that point. For those people who are afraid or, you know, don’t have the expertise of data yet of how to learn the language of data and speak the impact, basically. That’s my sort of passion, combining the two people and data and solve that problem for professionals.
Sylvie:
And how do you find because often, especially now, there’s so many HR tools. And of course we have, you know, so many tools. We can collect a lot of data. And big companies when they’re doing learning. Do you find the teams know that they have their problem? Like do they have a profile? Like I am working on learning design, therefore I’m sort of not a numbers person. I’m afraid. Or is it more a thing like, I have all this data and I think I’m analyzing it correctly. And your point is like you still need to question assumptions and look at it differently.
Zsolt:
There’s so much data, just like content, that we’re drowning in them. Now, the problem is that that doesn’t start your your process doesn’t start with technology. It doesn’t start with some sort of ROI calculation. It starts with thinking differently about data itself and why in the world you’re collecting, what you’re collecting, what are you trying to do with it?
What sort of, decisions that’s going to help you to do? And so it’s kind of like working backwards from, from the end. And so one of my sort of sayings all the time is think about data as a language rather than numbers on a spreadsheet and use a language. You actually have to say the right words and sometimes few words is off. The right way to the right people. Data itself is not going to be enough to convince people.
What’s going to convince people is your interpretation, your narrative, your story that you use. So this language. And so that was my challenge for a long time of how do I take spreadsheets and charts and stuff to people who are more likely inclined to be, you know, again, creative and maybe have of the very basics about data classification, but they’re very enthusiastic to use those.
Sylvie:
Right. And so this is how you develop this sort of workshop dev learn where you’re helping learning professionals go through this journey. So maybe we can talk a bit about that workshop, like what would happen. And maybe we’ll go into some examples of how we can look at data differently. The two of us right now, a couple use case.
Zsolt:
Yeah. So let’s pretend that you’re actually coming to Dublin, which is a conference coming up, in the fall. It’s actually the 20th anniversary. So we’re enthusiastic talk about that. Do that’s data again from. But what what’s going to happen? let me paint a picture of this. So imagine you are part of a team. You’re sitting around a table, with unknown people. That’s the workshop. You can, you know, anybody can join us. And about ten, 15 people the most sit at the table and in on the table, there’s actually a dashboard.
Now, it’s, it’s a printed, game board that looks like a dashboard, a typical, like, learning dashboard. So you see how many people completed the course, the ratio of dropping out. There is even numbers, scores, satisfaction rate, that sort of thing. And all they need to do by the end of the workshop is agree whether this pilot that represented on this, this dashboard was successful or not and that’s all that’s a very simple statement. What happens throughout this six hours, though, they realize that what it’s what seems to be a simple decision.
It’s more complex and more murky because they’re not going to even agree on what they see and how they interpret simple numbers, like, for example, a satisfaction rate of 4.4. You’ll see that they spend so much time arguing whether a simple statement about that number is true or false or unknown. That’s the whole workshop. They have to go through this understanding the charts. For example, one like we can pick up one of the charts, be a little game with you and chart one of how would you sort of approach that and see how that works?
Sylvie:
Okay. Yeah. Well so like on the so I’m looking yeah. It looks like a game. It’s like, you know, a card with a bunch of a bunch of numbers. And it’s true as you’re talking, it’s even resonating, you know, like just I’m thinking of our own dashboard that we use that Lynx, and we have different engagement metrics. But some courses the goal is completion, whereas some courses or the not of courses but the offer the goal is access. And so then we ourselves, we go up and back like why track engagement on an access metric if that’s it’s nice if they’re engaged.
But that wasn’t the point. So I mean I see how we go round and round. okay, so I’m looking at your dashboard. I see a bunch of metrics. And this is one okay, average five star rating of the satisfaction. And it’s 4.4. So what do we think about that.
Zsolt:
All right. So first of all I want to make clear definitely this workshop the six hours is not a evaluation. So the workshop when you walk out and you have an evaluation measurement and evaluation method, it is really down to the nitty gritty about how to use data and speak to data, and especially how to think about data. And so in this case, it’s not a it’s not a question about whether the ROI was XYZ, z. It’s about each number, each color, each everything on his dashboard.
And what does that mean? What’s behind them. So for example, 4.4, if I tell you that that the satisfaction rate on a Likert scale or Likert apparently how the guy, the last name is pronounced, 125 and you got, a couple of hundred responses and they average that together. So basically add them up divided by the number and they got 4.4.
There’s an asterisk on that as you see on that. on the, on the dashboard. And it says explaining that there was one single feedback that they excluded, which was a one star. Everything else was five, and four and three. And because it was about the, you know, not so someone complained about the internet connection in the open tech. So they know it wasn’t about the content. So they excluded that one. And that’s how they rounded up to 4.4, which was their target.
So they’re happy that they reached a target. So the first question is what they are right. Would you throw out one single item a feedback if it’s so so far out that’s this is one and everything else is about three, right.
Sylvie:
Yeah. On the one hand yeah. Would you, would I, I mean I see the both sides on the one hand. Yes. Because it’s an outlier. On the other hand, that person had a pretty bad experience. So you should include it okay.
Zsolt:
So that’s not that. It’s. Because again imagine that you have ten people in the room, in your table. And you have to make a decision whether you would do it or not. What is the it’s sort of unknown. So you would not, touch it. So one thing I always encourage people to think about in this way is what is the end result. So what I tried to do here and there are two things I want is the technology, and statistics.
So there are statistical ways to declare what an outlier is. And we’re not going to get into that today. But literally, you know, how far out from sort of the mean the middle. And you can use that to a before you even collect data, declare of of what are you going to do with outliers? So don’t ever do this afterwards.
Like, oh, this is low. So let’s get rid of it. I make up a rule that we had this in our strategy because what it is, is five. Would you get rid of it to know because that helps you to move up the thing. So what.
Sylvie:
It’s totally what we always this is completely what we always do. We always do.
Zsolt:
oh. Yeah. It’s like, hey, can we do some thinking about that number? it’s also, have a bigger problem here, which is the by the Likert scale. So let me ask you this. So yeah, you know how the Likert scale works. It’s like 1 to 5 and then they pick one number. The question is would you treat that number that they pick as a choice between 123, four five.
Or would you treat that number as something as a continuous scale from 1 to 5. And so they could pick any number right.
Sylvie:
Like I would think of it as my continuous scale.
Zsolt:
If you think about it as a continuous scale, you already have an assumption and it may work out if anybody else would do the same assumption. But here’s what happens. if you think that way, that’s a continuous scale. If I can pick any point on that scale, do you think we still pick that exact same spot for three?
Sylvie:
Right. Probably not.
Zsolt:
No. No that would be spots above and below three. So what you do now is actually introducing a rounding problem because you treat every number that’s close to three as three. Because I might put like a 3.4, 3.5 maybe, or even 2.6 when I chose three closest. So basically my thinking is it’s less than four, but more than two.
So when you round these you lose the basically the granularity of that number, even if they thought about it like a scale and worse, when you start adding these rounded numbers and then you do in 4.4, then I use rounded numbers for a mean. That’s probably the worst thing you can do with numbers.
Sylvie:
Yeah, you’re completely right. And so what do you I mean, in fact, every single event that I’ve ever gone to sends me a thing. And you click a number of what is your rating? And so, yeah. What is your conclusion on all these sorts of things. Like when you rate something that it’s not really meaningful, like you really have to be intentional about how you ask the question, how you organize it.
Zsolt:
So this is a problem that we normally have within I think the learning professionals is is oversimplifying things because there’s there’s so much there’s actually two camps, two very loud camps about this one. It says these are actually and there are data classifications term for these, but these are actually just simple choices. And and there’s an order.
So it’s an ordinal number like three and two. Four is bigger than three. But these are not continuous scale at all. And even the distance between them and not the same. So what I mean by that is do you think it’s the same if you think about a Likert 1 to 5, it’s the same effort to move from 1 to 2 or 4 to 5.
And we don’t know because it’s completely random. So if you’re the traditional camp, all you can do with this number technically is count them of how many you have not treat them as a number, and try to write math calculations on top of them. So you could say that 90% of the people picked four and five. Yeah.
And that’s it. That’s sort of your approach. Now if you’re in the new camp and say, hey, if there are certain circumstances you can use this A) you need sample items. Because the fewer items you have in this, the higher a chance that you have these outliers. And actually both means all over two. which is a problem with classes because it’s small.
Second is, there are tricks to do this, breaking it down, maybe into three questions. And then now you have a 3 – 15 scale rather than a 1 to 5. But then you have to deal with the problem of on those three questions, actually measuring a compound like a good construct for one thing. But now you can add so for example, engagement could be measured in three different ways.
Physical engagement, emotional engagement and then cognitive engagement. And then you sort of combine them in a scale. But you see how complicated this single number is. And on top of that, here’s my biggest problem with that. Don’t ever use this as a single decision making. But yes, you can use that on the chart somewhere along with other things.
So you can detect disasters. so what happens is that, you know, if you get a 2 suddenly that, you know, something went wrong. And so you can, you can, you know, your detective work, but by itself, this number should never be on a target. So you should not start with we have to have 4.4. It doesn’t make any sense I think.
Sylvie:
Yeah I thought of a lot of things. you were talking. Yeah. One that was your last point. Was one that occurred to me. It’s almost like set a benchmark and that you’re you decide the way you ask the question, the sample size. And you know, like, listen, when we do these workshops, we should get 90% of the people saying 4 or 5.
If all of a sudden, you know, we’re at 85%, something went wrong. And it should just cause you to look rather than, you know, you’re looking at a trend rather than, an absolute, and that also it’s it depends on so many things, like a learning program. Right. I think the internet example is actually interesting because I’ve sort of has nothing to do with what you’re focused on.
But you’re asking like, did you have a good experience actually was terrible. I was hot or I couldn’t connect or the sound was bad or whatever. And so that’s not a business impact, but it’s sort of like a valid a valid thing. So yeah.
Zsolt:
And that’s that’s exactly the point. So every time you see anything on a chart or you collect data for the first question is why?
What are you measuring and why, if you’re not going to make any decisions based on the numbers, whatever the result is, then why you’re measuring the whole thing is basically just waste of time. And if you do, then you need to be aware of what might be the traps. So in this case, if you start creating averages and a rounded problem, then okay, that’s maybe fine.
But a you should also see the, distribution of your responses. So when you plot them out, it should look like a normal, like a bell curve sort of thing. If you if you get the right sample size and it’s a naturally occurring sort of result, if they don’t look anything like that, then for example, I mean, doesn’t make sense.
It actually leads to a, which I have many examples in this dashboard. When we just look at the mean itself, it’s actually meaningless.
Sylvie:
And so how do participants like when they go through it. So this is just one number. And already my head is like spinning the thinking like, oh, I see how you could spend six hours trying to figure this out. Like, what is their takeaway? Did they say like it kind of in a panic like, oh no, how am I going to think about data? Or do they what like what is your recommendation to them. Like look at fewer data points, be intentional. Be more careful. Like how do you solve this, you know, math problem basically.
Zsolt:
So this is why I said at the beginning that this is not a workshop about, here’s the five things. In order of what you do to get an ROI number. This has nothing to do with numbers. Actually. It’s all about thinking. And my goal with the workshop as they come out is that they actually think very differently about data itself from the beginning, and that’s part of it. Again, if you think about it as a language, what sort of vocabulary I need first before I can talk.
So don’t just say things that some politicians do without any meaning behind them. Say as few words as you as you need to, but make your story heard and make your story, tell the impact to the right audience. And so what we’re focusing on mostly is this. It’s almost like this critical thinking part, but in, in contexts, in applications.
So here’s the number. What might be the things that contribute. The interpretation is how would others see that. So one takeaway that people have at the end is that they never thought that others looking at the same number the same way, and the same statement about that number, they would disagree, but not they can see that when they go back to their work and random people come in to discuss things now, they can anticipate those, like just because it’s, clear for me on this one chart or one number, it doesn’t mean that the same for everyone else.
And if there’s one thing that I can recommend, where to start sort of building this, thinking differently about data. That’s a great book. Jordan Morrow wrote, a couple of years ago. It’s called Be Data Literate. And literally that’s the title. So he goes through these three C’s, you know how to think critically and be creative sometimes with data.
And I also in my workshop added one more, which is, culture the 4th C because what, what I noticed also that general up the shelf of course is there’s many of them out there. The heart there are hard to translate into your own culture unless you know how to get things done internally. So you need to actually adopt all these, not just take something, buy something and run with it as you, as you, as you think you would do with other products.
Sylvie:
Yeah, no. It’s amazing. I love how you think about this. And actually it’s, you know, my background. I was a CFO for a long time, before I did what I’m doing now. And one thing I really liked about that was looking at all the financial data and saying, you know, what story does it tell? What does it mean?
What are the numbers that you know, how do we distill these numbers to tell a story and explain, you know, what’s happening in a business or with a project or with an investment or something? And it’s actually, I would say, a little easier with those kind of numbers because there’s there’s still interpretation, but less. But it’s amazing how with these HR metrics and especially around learning and how you can link, you know, how to start thinking about linking what you’re doing in L&D to performance outcomes to personal outcomes to development.
It’s actually yeah, really hard. And, you know, thinking critically about the data and how you’re going to analyze it is actually key before you start drawing a bunch of conclusions that actually make no sense.
Zsolt:
And speaking to that, once the team actually figures out about this dashboard, what’s what, they agree and they make those statement that is actually half of the workshop, then they switch to dashboards to the other side, and that’s the performance dashboard.
So now they can talk about correlations between the learning and performance. And that’s where things get interesting because it may seem like training is actually work well on the training side of metrics, but not so much for performance. And then now what? But I won’t tell you what the end result is because obviously you have to come to the workshop I know.
Sylvie:
Yeah. So tell us more. Tell us more about your workshop. How do we how do we find it? And how do people join up.
Zsolt:
So November I think fourth or also is doubler is a pre-conference workshop. It’s it’s a full day and then you can stay for the conference after if you, wanted to. And where? It’s, it’s in Las Vegas, almost. Vegas, Nevada in the States.
Sylvie:
Amazing. All right, well, I don’t often go to Las Vegas, but maybe this is my excuse. well, this is super, super interesting. And again, I’m, like, so inspired because, I think my personal opinion, it’s such a challenge in L&D in particular, of showing the business impact of what we’re doing.
Like, I genuinely feel that like investing in people, investing and learning is the most important thing a company can do, and yet it’s the easiest thing to first cut when budgets get cut. And I think, you know, if you can evangelize this and make it where we can actually show good data, understand what it means and where people believe, I think it can make a big difference in how a business perform. So fingers crossed.
Zsolt:
And the story you tell starts with, the story you imagine for yourselves. So if you stick to just learning data in your LMS, I don’t think many of the business leaders would care about your completions unless it’s like, you know, some sort of compliance thing. or the rate that they spend on certain activities and that sort of thing.
The hard part here is two things. One, show the impact and show what the impact was actually driven by learning. And that’s why if you just give up and go back to what can we do on our own so we don’t have to deal with others, across departments. And so like, oh, we have anonymous. Yeah. The numbers on the elements.
It’s a great start. That’s a good start. But it was a good start 20 years ago and we should really move move. So that’s by 2024.
Sylvie:
Yeah. So I’ll agree. Well thanks a lot. This was amazing. Thank you for joining us. And I’m going to follow carefully what you’re doing. And I’m going to read that book.
Zsolt:
Thank you so much Sylvie. Bye bye.
Sylvie:
Thanks for listening to this episode of “Talent is Everywhere”. Make sure to subscribe if you like what you heard and give us a follow on LinkedIn to continue the conversation on all things career mobility and talent development.
Is there a topic you’d love for us to cover in a future episode? Or a guest you’d recommend? Drop us an email at hello@lynxeducate.com
And if you’re looking for support on your talent development strategy, head over to www.lynxeducate.com to learn more about our career mobility solution. That’s “L”, “Y”, “N”, “X”, “educate”, “.com”.